报告题目:Sequence Maps — Sophisticated Accelerometer Data Analysis
报告人: 王新辉 研究员 阿姆斯特丹自由大学
照片:
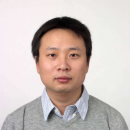
邀请人:刘振华
报告时间:2019年7月8日16:00-17:30
报告地点:南校区信远楼2区206报告厅
报告人简介:王新辉,男,博士,阿姆斯特丹自由大学研究员。2004年获得西安电子科技大学理学博士学位,2008年获得荷兰屯特大学第二个博士学位。先后在荷兰乌特勒支、内梅亨和阿姆斯特丹自由大学进行博士后研究。目前主要从事临床医学的多元组学分析和基因组学的分析研究,曾任二十几个期刊和会议审稿人,发表SCI 40余篇,他引600余次。
报告摘要:
To date, epidemiological studies have focused on the potential health effects of total volume of physical activity (PA) or sedentary behavior (SB). However, the total volume of PA or SB from different person might be similar, while but accumulated sequence might different. The pattern of PA and SB might play a more important role than the total volume to health and diseases. Therefore we aim was to develop a sophisticated algorithm translating accelerometer data into detailed sequence maps considering, and the machine learning methods, and other tools could be applied on this data analysis with this approach.
We developed a novel approach to transfer accelerometer counts into a sequence map based on behavior states defined by a combination of intensity (SB, light, moderate, and vigorous intensity) and duration (sporadic accumulation or in bouts of different duration). Additionally, hierarchical cluster analysis and statistical analysis were applied to identify performance of children with similar behavioral sequence maps.
Clustering resulted in seven clusters of children with similar PA and SB sequence maps: an average cluster (33% of children); a cluster with relatively more SB, light and moderate PA in bouts (SB and PA bouters, 31%); a cluster characterized by more sporadic SB and light PA (light activity breakers, 26%); and four smaller clusters with 7% of the children or less.
This novel algorithm is a next step in sophisticated analyses of accelerometer data with combination the intension and duration of original sequence. The sequencing was reduced with this approach, which could be used for machine learning method. The applications of this approach on many cohorts are presented.