报告题目:Algorithmic Design for Wasserstein Distributionally Learning
报告人:陈彩华 教授 南京大学
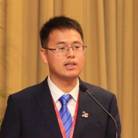
邀请人:赵志华
报告时间:2022年5月24日(周二)15:00-17:30
腾讯会议ID:434-637-536
报告人简介:陈彩华,南京大学理学博士,新加坡国立大学联合培养博士,曾赴新加坡国立大学、香港中文大学等学习与访问。主持/完成的基金包括国家自然科学基金青年项目、面上项目和优秀青年项目等,参与国家自然科学基金重点项目,代表作发表在《Mathematical Programming》,《SIAM Journal on Optimization》及CVPR、NIPS等国际知名学术期刊与会议,多篇论文入选ESI高被引论文。获华人数学家联盟最佳论文奖(2017、2018连续两年),中国运筹学会青年科技奖(2018),南京大学青年五四奖章(2019),入选首批南京大学仲英青年学者(全校10人,2018)及江苏省社科优青(2019)。
报告摘要:Wasserstein Distributionally Robust Stochastic Optimization (DRSO) is concerned with finding decisions that perform well on data that are drawn from the worst-case probability distribution within a Wasserstein ball centered at a certain nominal distribution. In recent years, it has been shown that various DRSO formulations of learning models admit tractable convex reformulations. However, most existing works propose to solve these convex reformulations by general-purpose solvers, which are not well-suited for tackling large-scale problems. In this talk, we focus on Wasserstein distributionally robust support vector machine (DRSVM) problems and logistic regression (DRLR) problems, and propose two novel first order algorithms to solve them. The updates in each iteration of these algorithms can be computed in a highly efficient manner. Our numerical results indicate that the proposed methods are orders of magnitude faster than the state-of-the-art, and the performance gap grows considerably as the problem size increases. Advanced models such as robust classification with fairness and unlabeled data are also discussed.
主办单位:best365亚洲版登录