报告题目:基于标签层次转移的分类模型
报告人:王仁振 西安交通大学助理教授
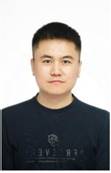
邀请人:贾西西
报告时间:2023年11月14日9:00-11:00
报告地点:南校区会议中心121
报告摘要:Hierarchical classification aims to sort the object into a hierarchical structure of categories. Existing methods commonly address hierarchicalclassification by decoupling it into a series of multi-class classification tasks. However, such a multi-task learning strategy fails to fullyexploit the correlation among various categories across different levels of the hierarchy. In this paper, we propose Label HierarchyTransition (LHT), a unified probabilistic framework based on deep learning, to address the challenges of hierarchical classification. TheLHT framework consists of a transition network and a confusion loss. The transition network focuses on explicitly learning the labelhierarchy transition matrices, which has the potential to effectively encode the underlying correlations embedded within classhierarchies. The confusion loss encourages the classification network to learn correlations across different label hierarchies duringtraining. We experimentwith a series of public benchmark datasets for hierarchical classification problems, and the results demonstrate the superiority of ourapproach beyond current state-of-the-art methods. Furthermore, we extend our proposed LHT framework to the skin lesion diagnosistask and validate its great potential in computer-aided diagnosis.
报告人简介:王仁振,西安交通大学best365亚洲版登录助理教授。研究方向机器学习、医学影像分析,尤其关注面向数据偏差的机器学习算法研究以及面向计算机辅助诊断系统的智能算法研究。目前在国内外权威期刊和会议上发表十余篇论文,包括人工智能顶级会议ICLR, ICCV, CVPR, MICCAI, IPMI及顶级期刊IEEE TPAMI, TMI及MedIA上。同时,担任人工智能顶刊IEEE TPAMI, TMI以及顶会ICML, NeurIPS, ICLR, MICCAI的审稿人。作为负责人主持国家自然科学基金青年项目、科技部国家重点研发计划子课题,并作为骨干参与国家自然科学基金重点及面上项目多项。